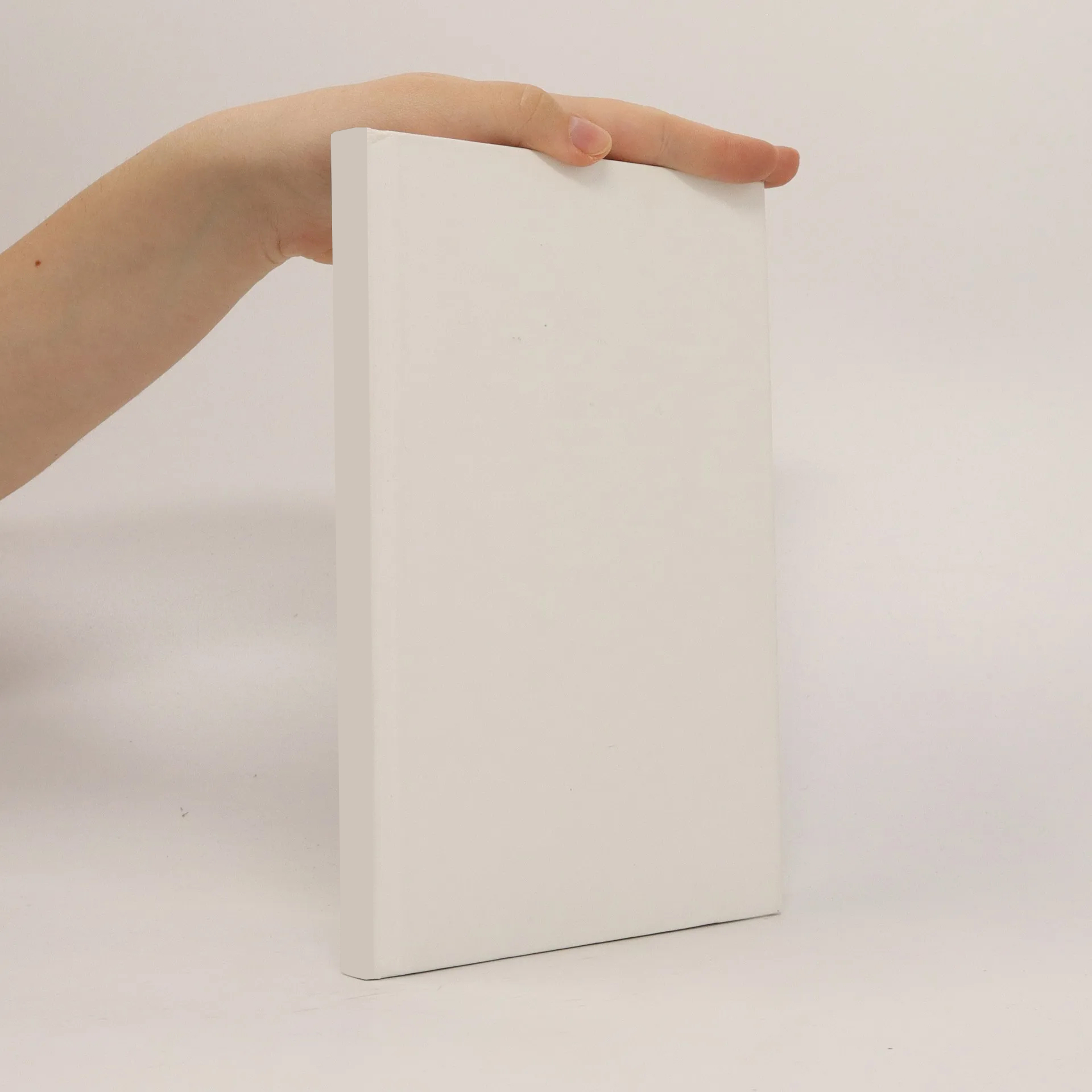
Viac o knihe
Recently, increasing interest has been shown in applying the concept of Pareto-optimality to machine learning, particularly inspired by the successful developments in evolutionary multi-objective optimization. It has been shown that the multi-objective approach to machine learning is particularly successful to improve the performance of the traditional single objective machine learning methods, to generate highly diverse multiple Pareto-optimal models for constructing ensembles models and, and to achieve a desired trade-off between accuracy and interpretability of neural networks or fuzzy systems. This monograph presents a selected collection of research work on multi-objective approach to machine learning, including multi-objective feature selection, multi-objective model selection in training multi-layer perceptrons, radial-basis-function networks, support vector machines, decision trees, and intelligent systems.
Nákup knihy
Multi-objective machine learning, Yaochu Jin
- Jazyk
- Rok vydania
- 2006
Doručenie
Platobné metódy
Tu nám chýba tvoja recenzia