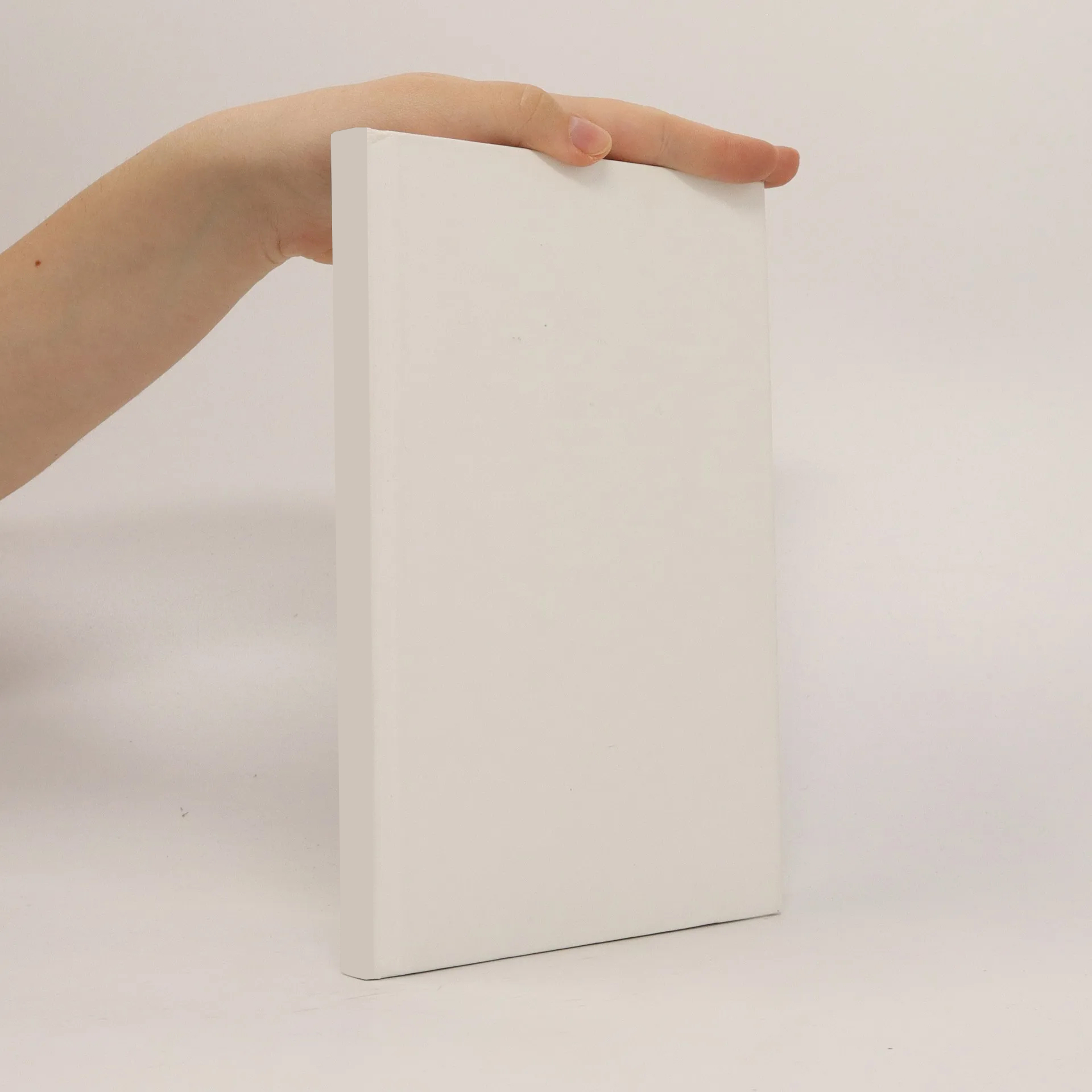
Off-board car diagnostics based on heterogeneous, highly imbalanced and high-dimensional data using machine learning techniques
Autori
Viac o knihe
Data-driven maintenance poses many challenges. Four very important of them, namely coping with a high dimensional and heterogeneos feature space, the highly imbalanced data sets, the Remaining Useful Lifetime (RUL) prediction of monitored parts based on short yet variable length timeseries, and already large but steadily further increasing data set size are identified. Each of the challenges is dealt with in one chapter. Novel techniques are designed, implemented, validated, and compared to existing approaches based on a variety of (publicly available) data sets for general applicability. In the following multiple concepts are proposed and evaluated in great detail: A feature selection pipeline with multiple consecutive stages of increasing run-time complexity but also increasing accuracy to tackle the high dimensional feature space. Existing techniques to tackle imbalance are evaluated and compared to a novel technique that stands out due to its extremely low computational complexity. Two novel techniques based on cascaded Random Forests (RFs) and on density-based estimation that outperform current state of the art techniques for RUL prediction. And finally: The evaluation of an in-memory cluster computing framework regarding its suitability for not only large-scale data set extraction from a relational database, preprocessing and transformation of the dataset but also machine learning.