Kniha momentálne nie je na sklade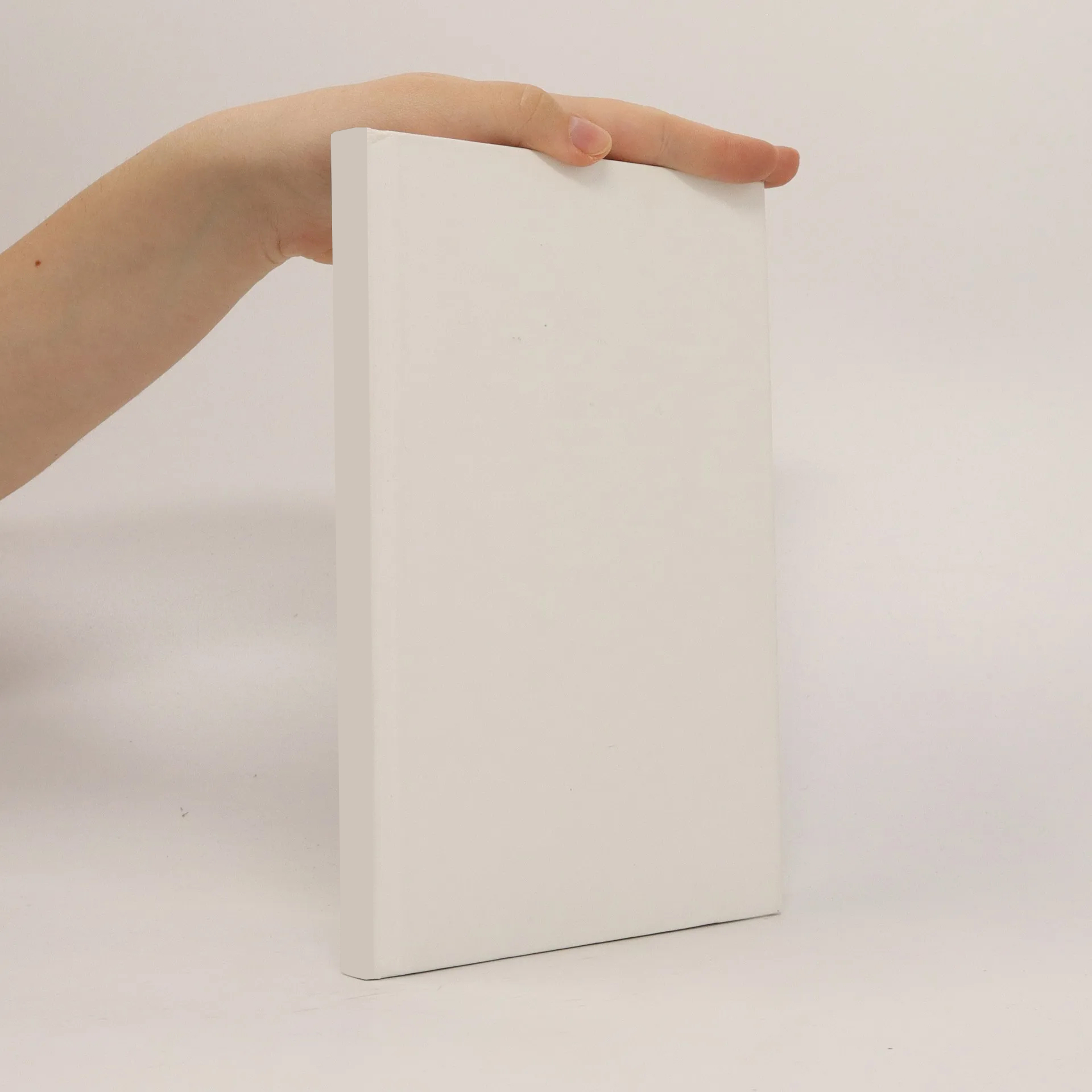
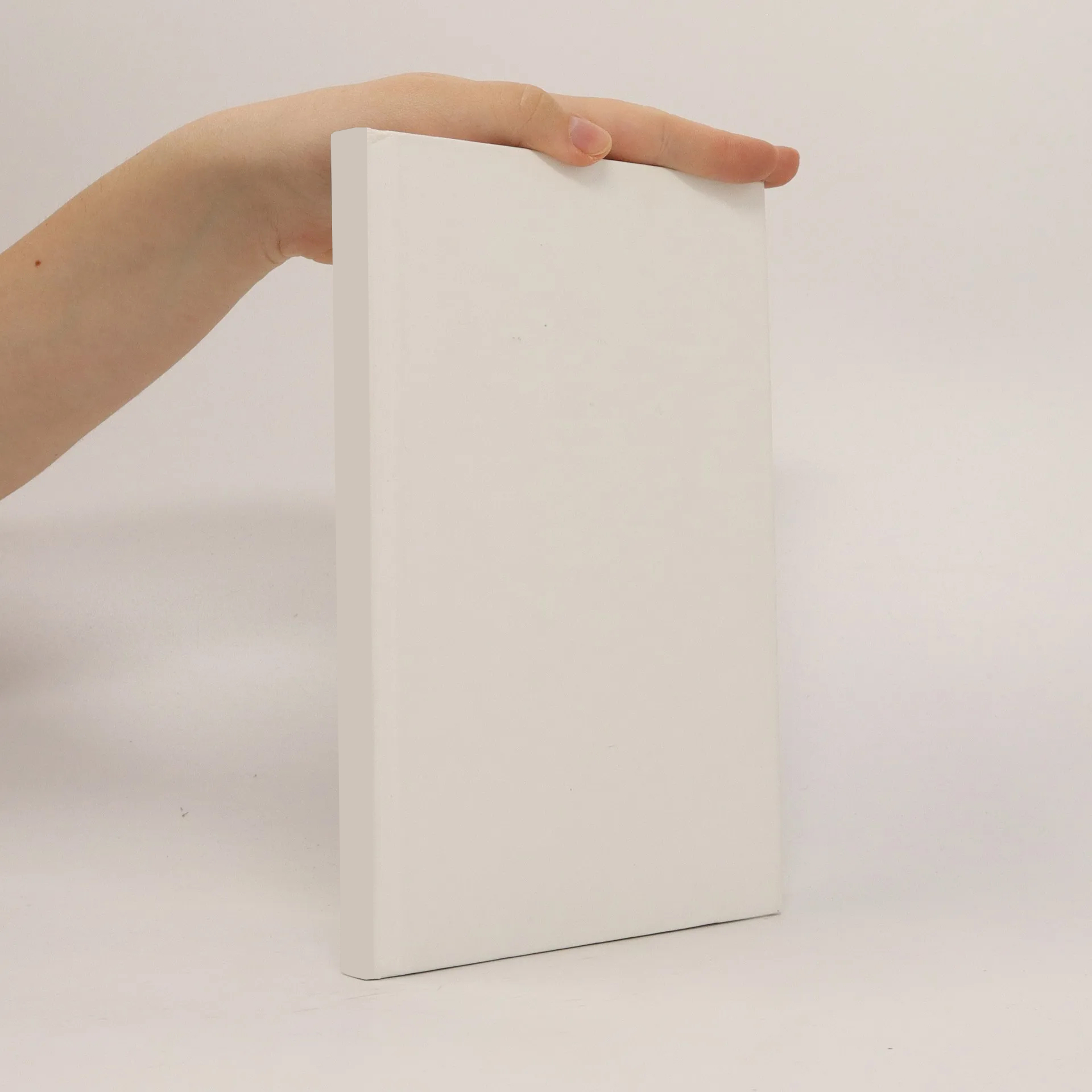
Viac o knihe
The book introduces a Markov chain approach for analyzing microscopic models of complex systems at the individual level. It establishes a framework for aggregation in agent-based models, utilizing lumpability and information theory to connect micro and macro observations. A detailed "micro-chain" representation is developed, focusing on transition rates and the stochastic elements that govern dynamics in "voter-like" models relevant to population genetics and social dynamics. The work emphasizes how aggregation issues in ABMs can be understood through a broader informational context, enhancing the analysis of complex systems.
Nákup knihy
Markov Chain Aggregation for Agent-Based Models, Sven Banisch
- Jazyk
- Rok vydania
- 2016
- product-detail.submit-box.info.binding
- (pevná)
Akonáhle sa objaví, pošleme vám e-mail.
Doručenie
Platobné metódy
Navrhnúť zmenu
- Titul
- Markov Chain Aggregation for Agent-Based Models
- Jazyk
- anglicky
- Autori
- Sven Banisch
- Vydavateľ
- Springer International Publishing
- Rok vydania
- 2016
- Väzba
- pevná
- Počet strán
- 212
- ISBN13
- 9783319248752
- Kategórie
- Matematika, Príroda všeobecne
- Anotácia
- The book introduces a Markov chain approach for analyzing microscopic models of complex systems at the individual level. It establishes a framework for aggregation in agent-based models, utilizing lumpability and information theory to connect micro and macro observations. A detailed "micro-chain" representation is developed, focusing on transition rates and the stochastic elements that govern dynamics in "voter-like" models relevant to population genetics and social dynamics. The work emphasizes how aggregation issues in ABMs can be understood through a broader informational context, enhancing the analysis of complex systems.