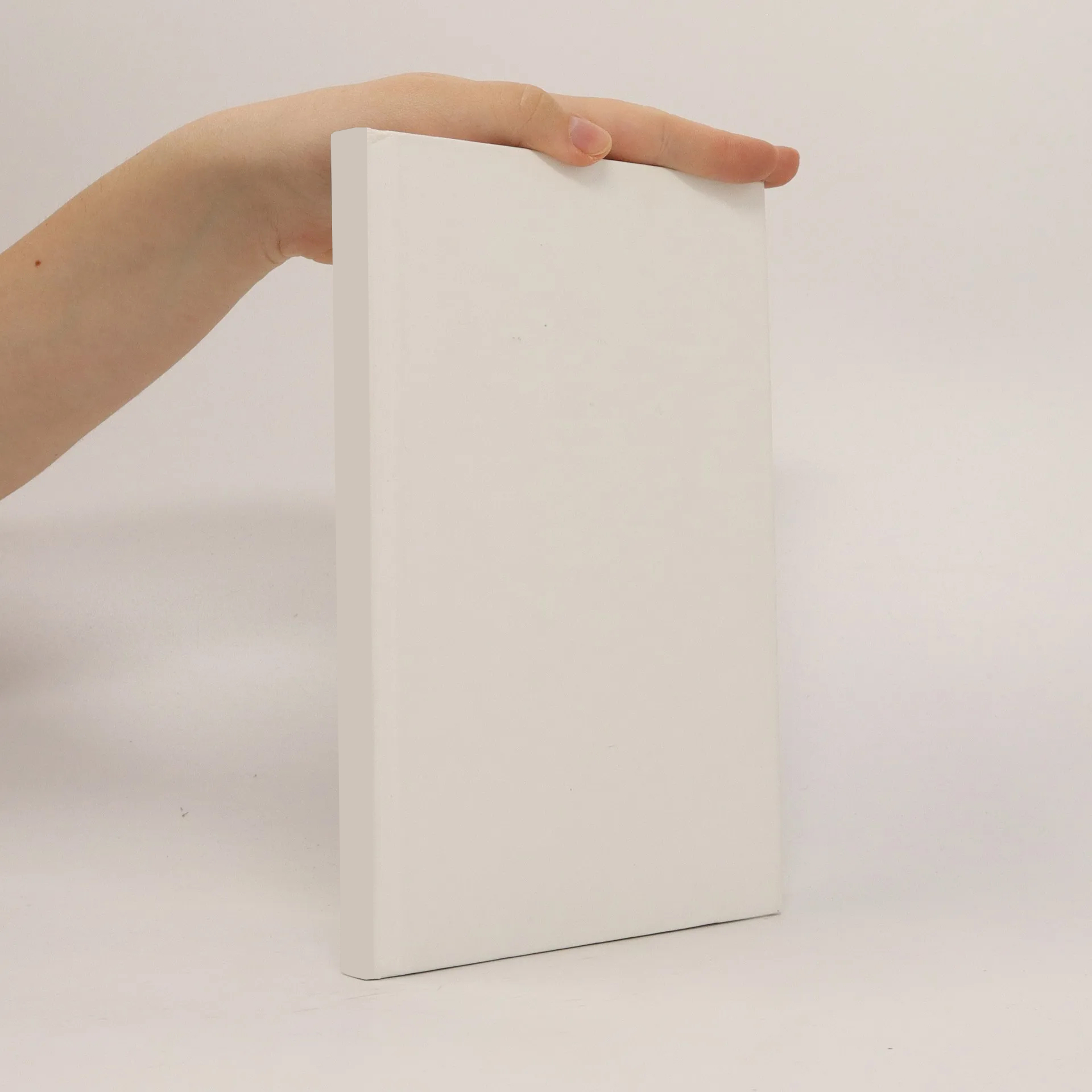
Multiple testing problems in the context of modern portfolio theory
Autori
Viac o knihe
A multiple testing problem occurs when two or more related null hypotheses are tested simultaneously. The classical significance level, which refers to the type I error of an individual test problem, is not suitable for testing a family of hypotheses. This is due to the fact that more than one true null hypothesis may erroneously be rejected. Several approaches to error control taking account of the multiplicity problem have been proposed. The focus of the present work is on the familywise error rate, i. e., the probability of rejecting at least one true null. The first major contribution of this study is the comparison of multiple testing procedures with regard to their robustness properties. Specifically, it is investigated whether a test procedure controls the familywise error rate under various data generating processes. In addition to error control, a robust multiple testing procedure provides reasonable power. The results of the simulation study suggest that using bootstrap methods to detect the dependence structure of the individual test statistics leads to improved multiple testing procedures. In the multiple testing literature, a distinction is made between all-pairwise comparisons, multiple comparisons with a control (MCC) and multiple comparisons with the best (MCB). The second contribution of this book constitutes the application of robust multiple testing procedures to MCC problems. Firstly, the effect of international diversification in the mutual funds industry is investigated by raising the question of whether emerging market funds are more likely to outperform the market than developed market funds. Secondly, we compare the performance of several portfolio strategies, which are derived within the Markowitz framework, with the performance of trivial strategies. The range of strategies includes the traditional sample-based approach, several minimum-variance techniques, a shrinkage, and a minimax approach. Three prominent performance measures are used in the empirical studies, including the Sharpe ratio, the Treynor ratio and the certainty equivalent. The third contribution is the derivation of the asymptotic distribution of pairwise performance differences under the assumption of (i) normal returns, (ii) i. i. d. returns and (iii) weakly dependent returns. Explicit formulas are provided for each of the three performance measures in order to calculate the asymptotic variances of the performance differences under the aforementioned data generating processes. The last part of the work deals with the specification of prior parameters in order to implement Bayesian approaches to portfolio optimization.